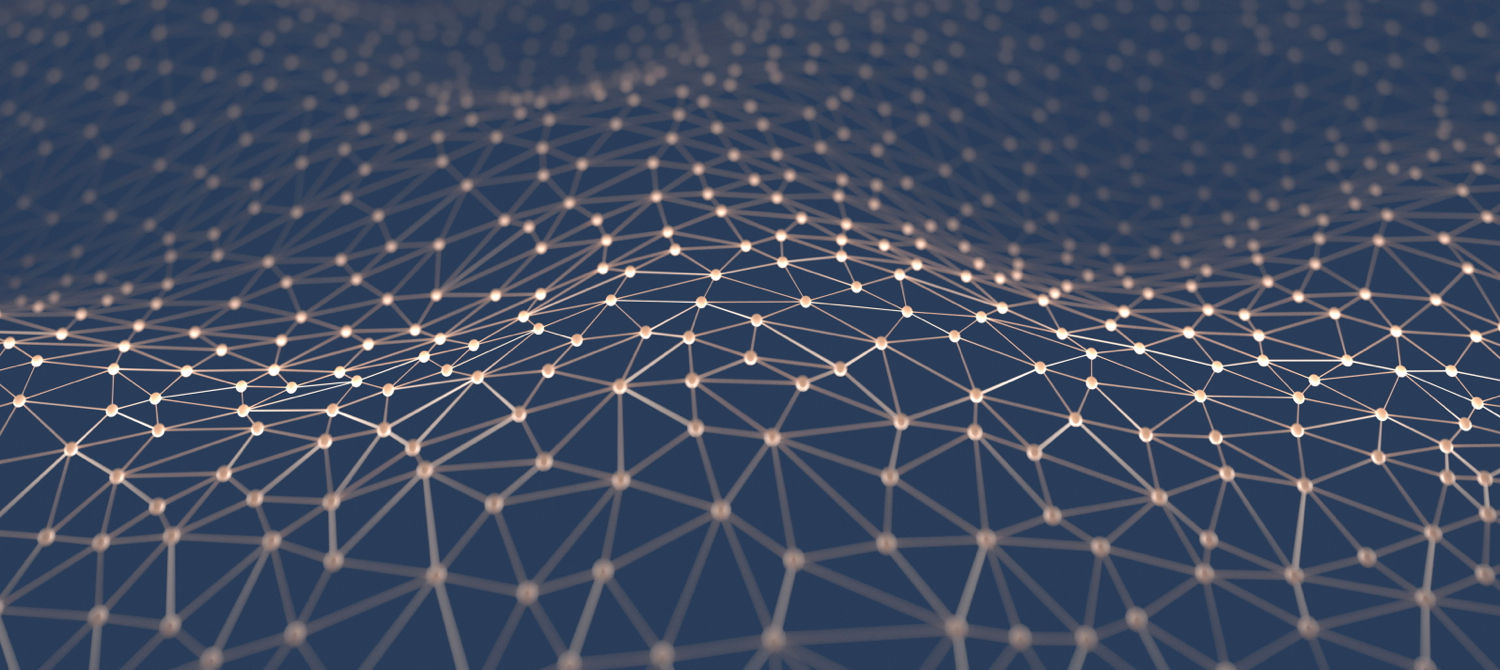
DEEP LEARNING, COMPUTER VISION, MEDICAL DATA ANALYSIS, ROBOTICS
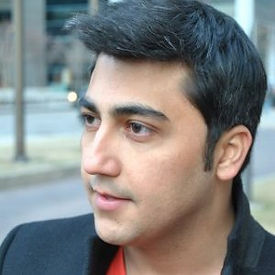
Jamal is currently a Data Scientist at the Corporate Research Lab. in 3M, St. Paul, MN. He received his Ph.D. in computer science from Michigan State University. His Ph.D. was focused on designing deep learning algorithms for medical image analysis. Jamal's research interests mainly lies at the intersection of computer vision and machine learning.
PhD Committee:
Dr. Arun Ross, Dr. Erik Shapiro, Dr. Xiaoming Liu, Dr. Hayder Radha
MOST RECENT AWARDS only
-
Best Industry Related Research Paper Award (runner up) in the 22nd International Conference on Pattern Recognition (ICPR-2014): Only, four papers out of about 1000 research papers are nominated for this prestigious award. His research paper proposes a novel feature representation called Histogram of Importances (HoI).
​
-
DewGood Award for Public Service in Technology (Honorable mention, 2015). Research: Automatic in vivo cell detection in MRI. His research is the first to show that stem cells can be detected in live organs by designing intelligent machine learning and computer vision algorithms operating on MRI. This research can significantly impact how diseases such as cancer, organ failures etc. are treated.
​
-
Recognition of Outstanding Research Award by Michigan State University (2015, 2016 - consecutively 2 years) This research focuses on how novel transfer learning approaches, via deep convolutional neural networks, be utilized effectively in the medical domain where there is scarcity of labeled data.


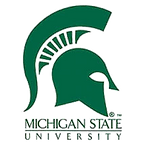
DEEP LEARNING FRAMEWORK FOR EXPLOITING SIDE INFORMATION
Application: Automated Cell Detection in MRI

This research investigate if the labeling behavior of an expert can also be exploited in addition to the conventional data and labels. There are three main challenges: (1) Unlike traditional features, labeling behavior (labeling latency) is only available in training and not in testing, (2) Unlike other side information, labeling behavior may only be available for 1 class, (3) Convolutional neural networks (CNNs) usually learn from image data and not from non-image side information. The proposed approach address all these challenges.
Muhammad Jamal Afridi, Arun Ross and Erik Shapiro “ L-CNN: Exploiting Labeling Latency in a CNN Learning framework” Proc. of 23rd International Conference on Pattern Recognition (ICPR), (Cancun, Mexico), December 2016. (To Appear).
SUPERFERN FEATURES AND ENSEMBLE LEARNING WITH BAYESIAN NETWORKS
Application: Automated Cell Detection in MRI
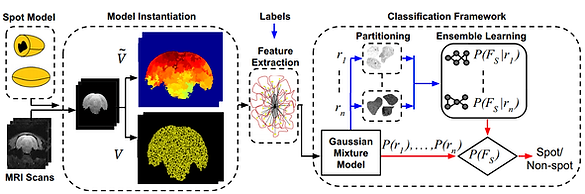
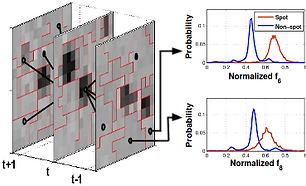
This research proposes a new set of features for cell detection in MRI, named as, 'Superferns'. These unlike traditional fern features, can exploit a superpixel based representation. The study also involves segmenting brain from MRI and oversegmentation via entropy based superpixels. A partition based ensemble of bayesian networks is utilized for learning classifiers.
Muhammad Jamal Afridi, Xiaoming Liu, Erik M Shapiro, and Arun Ross, “Automatic in vivo Cell Detection in MRI,” in Proc. 18th International Conference on Medical Image Computing and Computer Assisted Intervention (MICCAI), Munich, Germany, October 5-9, 2015.
HISTOGRAM OF IMPORTANCES: GLOBAL SUPERPIXEL BASED IMAGE DISCRIPTOR
Application: Plant-level Disease Rating in Real Agricultural Fields

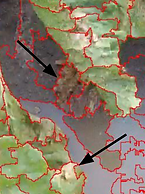
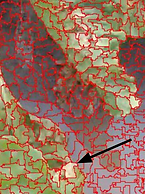
This research proposes a new set of features - Histogram of Importances - that allows local superpixel features to be utilized as a global discriptor for the image. In this project, these features were utilized to conduct the first research on automatically rating diseases in plant level images obtained using a camera on field tractor on real agricultural fields. The sysetm performed well on the data obtained for a period of several months. The results were exciting and the corresponding conference paper was nominated for the best industry related research award at the ICPR-2014.
Muhammad Jamal Afridi, Xiaoming Liu, J. Mitchell McGrath, "An Automated System for Plant-level Disease Rating in Real Fields," in Proceedings of the 22nd International Conference on Pattern Recognition (ICPR 2014), Stockholm, Sweden, August 24-28, 2014 (BIRPA Award runner up)
Yousef Atoum, Muhammad Jamal Afridi, Xiaoming Liu, J. Mitchell McGrath, Linda E. Hanson " On Developing and Enhancing Plant-Level Disease Rating Systems in Real Fields"
Pattern Recognition, Vol. , pp., Dec. 2015
MACHINE LEARNING FOR eHEALTH
OG-MINER: An Intelligent Health Tool for Remote Patient Monitoring Systems
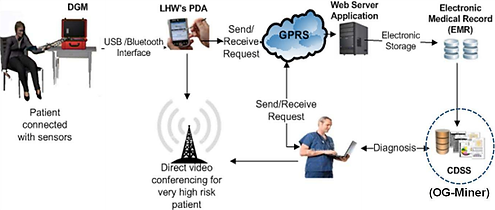
Muhammad Jamal Afridi and Muddassar Farooq, "OG-Miner: an Intelligent Health tool for achieving Millenium development goals in mHealth environments", Published at 44th Hawaii International Conference on System Sciences (HICSS), Hawaii, USA 2010. (Oral)
BASIC UNDERGRADUATE PROJECTS
Autonomous driving and robotics